By Stuart Rose
Today’s insurers are using analytics throughout the business to improve performance and reduce risk.
Insurance relies on the ability to predict future claims or loss exposure based on historical information and experience. However, insurers face an uncertain future due to spiraling operational costs, escalating regulatory pressures, increasing competition and rising claim expenses.
More than ever, insurance companies need to optimize their business processes. But what does that mean in practice?
Today, predictive modeling and forecasting are used by actuaries for pricing, but few insurance companies have applied analytics in real-time or near-real-time operational environments.
The “analytical insurer” is an insurance company using analytics throughout its organization to improve business performance. It has five major components:
- Actuarial analytics
- Claims analytics
- Customer analytics
- Channel analytics
- Risk analytics
Actuarial Analytics
The essential key to success and maximizing revenue is the ability to price your products appropriately, and insurance is no exception. A fundamental problem in actuarial science concerns rate setting, or the pricing of each policy. The goal is to set rates that reflect the risk level of the policyholder by establishing the break-even rate (premium) for the policy. In other words, the lower the risk, the lower the rate.
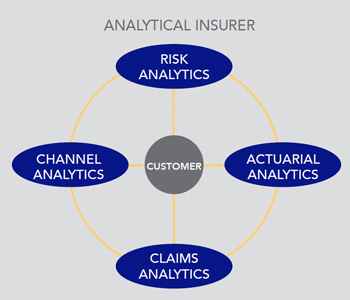
To gain a competitive advantage, insurance companies have been mining data to find surrogates that reveal information about the insurable risk. In the past, actuaries have heavily relied on univariate or one-way analysis for pricing and monitoring price efficiency. Today more and more insurance companies are using multivariate statistical techniques like generalized linear modeling for pricing to develop more accurate pricing structures.
The critical question in rate making is: “What are the risk factors or variables that are important for predicting the likelihood and severity of a loss?” For example, in the automobile insurance industry, a significant, positive correlation exists between the likelihood of a claim and the policyholder’s closeness to a large urban area. Actuaries might use this knowledge to specify automobile rates by postal codes for a given policyholder profile. As a result, a 30-year-old male who has been convicted of one driving violation in the past three years is likely to pay a higher rate if he lived and drove in a large urban area. Although many risk factors that affect rates are obvious, subtle and nonintuitive relationships can exist among variables that are difficult, if not impossible, to identify without applying more sophisticated analyses. Modern data mining models can more accurately predict risk; therefore, insurance companies can set rates more accurately, which in turn results in lower costs and greater profits.
Data is fundamental to any actuarial analysis, and the amount of available data is growing at exponential rates. While data quality is often cited as a challenge for accurate pricing, so is data accessibility. Often actuaries have relied on using just a subset of historical data to run their pricing models, since the time taken to prepare the data and run the appropriate analytical models is too long. To combat this problem, insurers are turning to in-database and high-performance analytics to provide faster processing on growing volumes of data.
Advances in technology have dramatically increased the amount and type of data available to insurers to build pricing models. Today, some insurers are incorporating data from credit scoring, social media (e.g., Facebook and Twitter), telematics from in-car data recording devices, and geospatial information (like Google maps) in their pricing models. Ultimately, however, there is only one rule with actuarial analysis: “An underpriced risk is the only bad risk.” And sending underpriced risks to your competitors is an outstanding method of providing you with a competitive advantage.
Claims Analytics
Claims disbursements and loss-adjustment expenses are by far the biggest expenses within a P&C insurance company and can be as much as 80 percent of an insurer’s written premium. The way an insurance company manages the claims process is fundamental to its profits and long-term sustainability.
Claims analytics is the process of analyzing the structured and unstructured data (i.e., email, adjuster notes, and medical records or police reports) at all stages in the claims cycle. Since up to three-quarters of claims data is unstructured data, the ability to analyze it is essential to improving the claims cycle.
Claims fraud is already a widespread problem for insurers, and in a difficult economy it tends to accelerate. The most effective way to combat both opportunistic and organized claims fraud is to use a combination of business rules, predictive modeling, anomaly detection and social network analysis. Using analytics will not only detect, but also prevent fraud before claims are paid.
No-fault fraud and abuse cost consumers and insurers approximately $204 million in 2010 in New York State alone, according to the Insurance Information Institute. Insurers often receive only a fraction of not-at-fault settlement costs because they don’t pursue subrogation opportunities. Using claims analytics, insurance companies can identify known subrogation characteristics and optimize associated activities.
Finally, some insurers are beginning to use analytics to calculate a litigation propensity score. Claims that involve an attorney often double the settlement amount and significantly increase an insurer’s expenses. Analytics can help insurers determine which claims are likely to result in litigation and assign those claims to more senior adjusters, who can settle the claims sooner and for lower amounts.
Customer Analytics
With little differentiation between product offerings, it is extremely challenging for insurance companies to retain customers, resulting in poor loyalty levels and increased acquisition costs.
Customer analytics is the ability to segment customers according to their likely buying behavior and potential profitability.
As the cost of acquiring new customers continues to rise, insurance companies are using analytics to develop customer retention programs. Data mining techniques can be used to predict the likelihood that a policyholder may not renew or may allow his or her policies to lapse. By proactively using this information, insurers can create marketing campaigns to prevent policy cancellation.
Another concept that insurance companies are beginning to embrace is the use of customer lifetime value. Insurance companies calculate the net present value of the customer based over an extended period, say five to 10 years. Knowing the lifetime value of a customer enables companies to benchmark how much they would or should be willing to invest to acquire/retain a customer.
Channel Analytics
The way that people buy insurance is rapidly changing. As insurance companies begin to implement multichannel integration strategies, the companies’ challenge becomes determining the right distribution method for each customer.
Channel management is certainly not a new concept; however, management decisions have traditionally been based on historical, periodic performance reporting. Product managers and field marketing can only react once an agent persistency rate or other KPI falls below an unacceptable rate, well after corrective action should have been taken. Channel analytics help to conduct “deep dives” into causal factors, which in turn require access to predictive data and forward-looking analyses.
Risk Analytics
The core business of insurance is managing and carrying risk, but many departments in insurance companies continue to operate as disparate units, which fosters a degree of uncertainty when it comes to understanding their business risk throughout the organization. In addition, the insurance company must effectively monitor its other risks, such as market, counterparty default, and operational and liquidity risk.
All of these risks should be managed through an enterprise-wide framework that allows the insurer to identify, measure, manage, report and monitor risks, and then adjust the company’s risk profile in line with its business objectives and risk appetite. There must be clear governance so that all employees—from the supervisory board and executive management to heads of business and compliance—know their responsibilities and the reporting procedures for noncompliance.
Effective enterprise risk management is about embedding risk management into everyday processes like finance, business planning and pricing at all levels of the organization. This requires unified, quantitative software that can provide integrated, comprehensive data management; powerful predictive analytics; user-friendly, self-service reporting; and a transparent environment that lets the risk professionals manage the entire process.
Conclusion
In a highly competitive market, it is vital for insurance companies to minimize inefficiencies and reduce losses to protect profitability. By using data proactively, companies can better understand their business, detect areas for improvement and take remedial action.
Analytics has become fundamental for insurers to remain competitive.
Stuart Rose is Global Insurance Marketing Director at SAS. He can be reached at Stuart.Rose@sas.com or follow him on Twitter @stuartdrose.